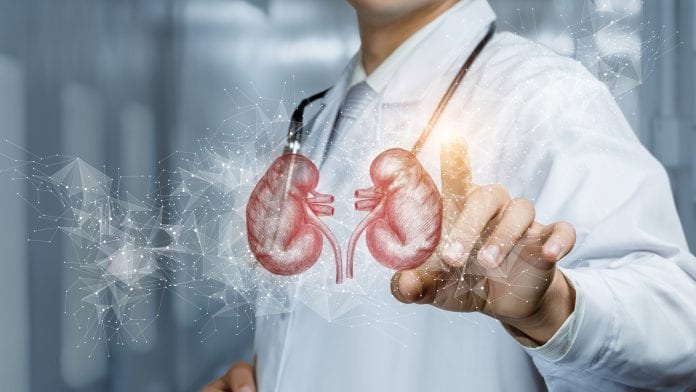
Researchers have utilised artificial Intelligence (AI) to help determine the extent of the damage caused by chronic kidney disease in patients.
A team of researchers from Boston University school of Medicine (BUSM) has developed a new AI tool that is able to determine the severity of chronic kidney disease (CKD) by predicting the grade of interstitial fibrosis and tubular atrophy (IFTA), a known structural correlate of progressive and chronic kidney disease.
Currently, CKD damage is assessed by scoring the amount of IFTA in a renal biopsy sample. Although the digitisation of images, along with morphometric (measuring external shapes and dimensions) techniques, can better quantify the extent of histologic damage, a more widely applicable way to classify kidney disease severity is needed.
Utilising AI
Corresponding author Vijaya B. Kolachalama, PhD, assistant professor of medicine at BUSM, explained that having a computer model that can mimic an expert pathologist’s workflow and assess disease grade is an exciting idea as the technology has the potential to increase efficiency in clinical practices.
Currently, typical workflow by the pathologist on the microscope involves manual operations such as panning and zooming in and out of specific regions on the slide to evaluate various aspects of the pathology. In the ‘zoom out’ assessment, pathologists review the entire slide and perform ‘global’ evaluation of the kidney core, and, in the ‘zoom in’ assessment, they perform in-depth, microscopic evaluation of ‘local’ pathology in the regions of interest.
An international team of five practicing nephropathologists independently determined IFTA scores on the same set of digitised human kidney biopsies using a web-based software. The team took these average scores and used them as a reference estimate to build the deep learning model.
To emulate the nephropathologist’s approach to grading the biopsy slides under a microscope, the researchers used AI to incorporate patterns and features from sub-regions of the digitised kidney biopsy image as well as the entire digitised image to quantify the extent of IFTA – creating a deep learning model was designed to accurately predict IFTA grade.
Kolachalama said: “Eventually, it may be possible to use this algorithm to study other organ-specific pathologies focused on evaluating fibrosis. Such methods may hold the potential to give more reproducible IFTA readings than readings by nephropathologists.”