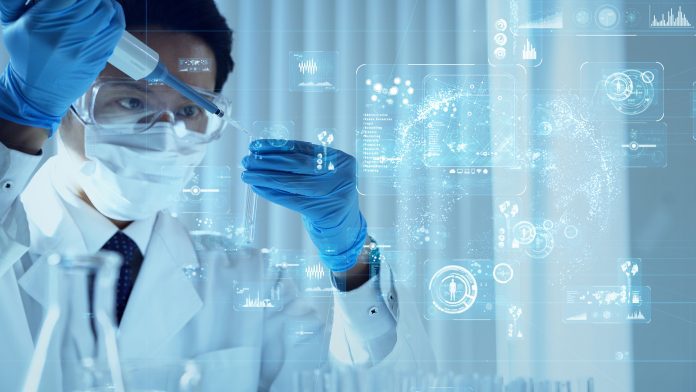
Artificial Intelligence (AI) can potentially reduce the cost and speed-up drug discovery, according to researchers at the University of Sheffield and AstraZeneca.
The new AI technology, developed by Professor Haiping Lu and his PhD student Peizhen Bai from Sheffield’s Department of Computer Science, with Dr Filip Miljković and Dr Bino John from AstraZeneca, could be the future of drug discovery.
The technology, named DrugBAN, can predict whether a candidate drug will interact with its intended target protein molecules inside the human body.
The study is published in Nature Machine Intelligence.
The current capabilities of AI in medicine
Currently, AI can predict whether drugs will reach their intended targets, but the new technology by the teams from Sheffield and AstraZeneca can do this with greater accuracy and also provides useful insights to help scientists understand how drugs engage with their protein partners at a molecular level.
AI has the potential to inform whether a drug will successfully engage an intended cancer-related protein, or whether a candidate drug will bind to unintended targets in the body and lead to undesirable side effects for patients.
The new AI technology is trained to learn the substructures of proteins in the human body as well as those of drug compounds. The technology then learns how these substructures interact, which it draws on to make predictions on how new medicines are likely to behave.
Haiping Lu, Professor of Machine Learning at the University of Sheffield, said: “We designed the AI with two primary objectives. Firstly, we want the AI to capture how drugs interact with their targets at a finer scale, as this could provide useful biological insights to help researchers understand these interactions on a molecular level. Secondly, we want the tool to be able to predict what these interactions will be with new drugs or targets to help accelerate the overall prediction process. The study we’ve published today shows our AI model does both of these.”
Furthermore, the AI technology learns pairwise substructure interactions, which is where multiple interactions take place between the substructures of drug compounds and proteins in the body. Most existing drug prediction AI tools learn whole representations of drugs and proteins, which don’t capture their substructures and therefore, provide less useful insights.
The team plan to use more in-depth data on the structure of compounds and proteins to make the AI even more accurate.
Improving drug discovery with AI
Drug discovery and development using traditional methods remains a difficult process as a result of lengthy development times and the need for large amounts of funding. However, drug discovery processes can be significantly accelerated using AI and digital technology.
Nick Brown, Head of Imaging and Data Analytics, CPSS, AstraZeneca, said “I am really excited to see this paper, particularly because, unlike other approaches, DrugBAN simultaneously learns from candidate drugs and their targets using a bilinear attention network, and is explicitly designed to generalise the problem.”
Professor Guy Brown, Head of the University of Sheffield’s Department of Computer Science, added: “Our research at Sheffield is strongly motivated by a desire to make a positive difference to people’s lives, and we see interaction with industry leaders such as AstraZeneca as crucial to that mission.
“This is exciting research which will hopefully allow significant advances in the design of therapeutics. The approach is also distinctive for its focus on interpretability, enabling human experts to benefit from insights generated by the AI system.”