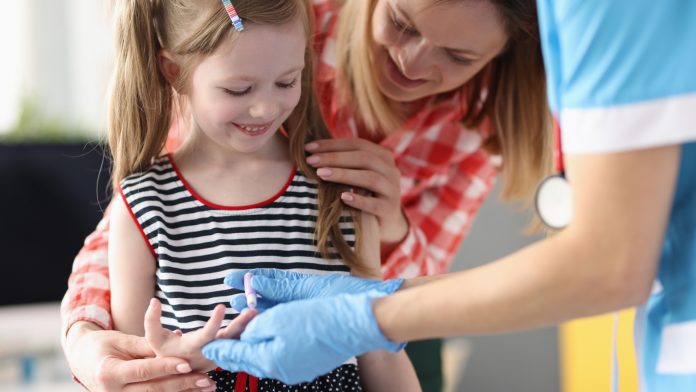
The PREcisE project draws together research to tackle the mother-to-child transmission of impaired glucose metabolism.
The prevalence of comorbidities associated with adult type 2 diabetes (T2D) continues to rise. T2D threatens the economies of all nations, particularly developing countries, and is robustly associated with the increased prevalence of childhood obesity¹. Mounting evidence has established that the risk for impaired glycaemic health in the adults starts at (pre)conception and builds up from one generation to the next. In addition to traditional environmental, social and genetic risk factors, the emergence of trans-generational effects suggests that part of the individual risk for impaired glycaemic health is carried out by epigenetic mechanisms. DNA methylation is one of the most studied epigenetic mechanisms and may mediate the impact of environmental factors on gene expression.
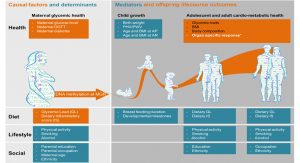
Paralleling the global epidemic of obesity and T2D, the prevalence of gestational diabetes mellitus (GDM) has been rising over the last decades. Women with GDM are at multi-fold risk of developing T2D later in life than women who did not have GDM. Moreover, there is a growing body of evidence that intrauterine exposure to GDM is associated with excessive weight and poorer metabolic health in the offspring already early in life². It has been hypothesised that the exposure to GDM alone might directly influence the foetal epigenome, which in turn may mediate the health of the offspring in later life.
The epigenetic theory has been tested in various experimental contexts including in animals and large-scale meta-analysis. Nevertheless, up until now the body of evidence to support a direct effect of GDM upon the child epigenome at birth remains contradictory.
Recent studies have reported DNA methylation marks in the cord blood of newborns whose mothers had GDM along the gene networks involved in metabolism³. Alongside the importance of confirming these findings in a large enough sample to account for essential confounding factors (Fig. 1), critical knowledge gaps must be filled to assess if DNA methylation may be used as a marker for personalised prevention by lifestyle intervention and precision nutrition.
Knowledge gap 1: maternal glucose as an underlying factor
GDM diagnosis is based on a clinical definition where the ‘dose-response’ relationship needs better understanding. Evidence from a Mendelian randomisation (MR) study as a joint effort by the Early Growth Genetic consortium suggests a dose-dependent effect of maternal glucose on infant birth weight4. This is also corroborated by observational studies on pregnancy complications, birth weight and cardiometabolic health in adulthood 5-8. A systematic investigation of the influence and causality of the dose-response relationship between maternal glucose and the child epigenome is still lacking, including a detailed analysis of the role played by psychosocial factors. Maternal body mass index (BMI), weight gain during pregnancy, age, lifestyle factors, marital status, and socioeconomic and cultural background could all be important factors to take into account, as they also contribute to the risk of GDM and offer an opportunity to take precautionary actions through healthy lifestyle choices.
Knowledge gap 2: the role of nutrition as a causal and/or modulating factor
Unfavourable dietary behaviour before and during pregnancy has been identified as a key risk factor for GDM, including dietary patterns associated with higher glycaemic response and/or inflammation9. There is evidence that a low glycaemic index (GI) diet improves glycaemic control in women with GDM10. Dietary behaviour during pregnancy not only affects maternal health, but also that of the offspring. The epigenome could be a prime candidate as the mediator of these relationships11.
Moreover, some of the epigenetic signatures identified at birth are expected to be reversible. Early postnatal dietary exposures, such as breastfeeding, have been reported to modulate DNA methylation at targeted loci12-13. Studies of monozygotic twins revealed epigenomic differences between twins: these differences were greater between older twin pairs with different lifestyles14, indicating that dietary and lifestyle factors also during later life may be important modulators of the epigenome. To address this gap, PREcisE is investigating whether dietary patterns modulate maternal glucose response (MGR) and later glycaemic health. These analyses have been made possible by of extremely well-defined dietary data and follow-ups.
Knowledge gap 3: tissue specificity
Many epigenetic mechanisms are in place to silence or activate the genes which determine the eventual fate of the body’s cells. This results in clearly distinguishable epigenetic patterns in each cell type15-16. It has been shown that, although DNA methylation signals were discovered in an epigenome-wide association study (EWAS) using whole blood samples, the trait-specific DNA methylation signature can be tracked down to relevant tissues17. For example, a BMI-specific DNA methylation signature discovered in whole blood was likewise observed in liver and adipose tissue18.
To address the gap in understanding of mother-child transmission of glycaemic health, PREcisE is conducting in-depth analyses of maternal glucose response and will follow up the signals in different tissues in our databases and others available for scientific research. This will allow us to assess a possible DNA methylation mediated effect of hyperglycaemic environment in utero on adult clinically relevant phenotypes in a tissue-specific manner, and to narrow down the most informative tissue for further analysis and experiments.
Knowledge gap 4: causality and reversibility
We will synthesise evidence from multiple sources to arrive at an informed judgment of causality. Currently, there is a vast debate about causality assessment and inference. Even methods that are widely considered to be a ‘gold standard’, such as randomised controlled trials, are not perfect19. The MR has recently been used extensively, and has been shown to be capable of tackling potential confounding factors and reverse causality. However, there are serious caveats because of often weak genetic instruments or pleiotropy.
It is evident that multiple epidemiological approaches should be applied to distil potential causal associations, but this more holistic view is often ignored. We could fill in this gap by seeking evidence from multiple high-quality studies, and by integrating diverse types of evidence and diverse strategies to assess causality, in order to rule out alternatives. These include MR approaches with genetic instruments (using both individual-level data and public databases for scientists); causal life-course models; negative/positive control approach; critical assessment on whether potential confounders or biases exist; and tissue-level pathway analyses using expression data. Fig. 2 shows the schematic presentation of potential life course model that incorporates both genetic, epigenetic, metabolic, clinical and lifestyle data, as well as socioeconomic information.
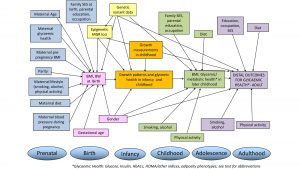
The PREcisE consortium
The PREcisE project was set up to answer the Joint Programming Initiative – A Healthy Diet for a Healthy Life (JPI-HDHL), calling for a better understanding of the causative mechanisms linking diet modifications and the human epigenome. The PREcisE consortium intends to perform its research through the use of data and biological atlas collected prospectively and harmonised in many European projects – the use of existing data is described below (Fig. 3). The analyses piloted in PREcisE can be extended by active collaboration with the Pregnancy and Child Epigenetic (PACE), and other consortia such as the GlobalMeth eQTL consortium.
Existing data
First generation birth cohorts
- The Northern Finland Birth Cohorts (NFBC) 1966 and 1986 are two large cohorts from early pregnancy, following offspring and their parents from year 1966 and 1985 to 1986 respectively. Details at www.oulu.fi/nfbc/;
- The Dutch Generation R study is a population-based prospective cohort study from fetal life until young adulthood, which enrolled mothers with delivery dates between April 2002 and January 2006, www.generationr.nl;
- The HAVEN is a study of children with and without congenital birth defects, focusing on maternal diet and one-carbon metabolism; and consists of measurements taken around 17 months postpartum of mother and child as a proxy of the periconceptional environment (https://doi.org/10.1007/s10654-014-9925-0);
- The Postpartum Outcomes in Women with Gestational Diabetes and their Offspring (POGO) study followed women diagnosed with GDM in Munich, Germany, during at least one pregnancy between 1998 and 2009, and subsequently their offspring (https://europepmc.org/article/PMC/3932071, DOI: 10.1900/RDS.2013.10.49; PMID: 24172698);
- A German prospective GDM study enrolled mothers with GDM and their offspring in Germany at birth between 1989 and 1999 (https://care.diabetesjournals.org/content/33/8/1845.long, DOI: 10.2337/dc10-0139; PMID: 20435793; PMCID: PMC2909075);
- The BABYDIET study is a dietary intervention study in newborns with at least one first-degree relative with type 1 diabetes (T1D) recruited Germany-wide between 2000 and 2006 (https://clinicaltrials.gov/ct2/show/NCT01115621); and
- The TEENDIAB study is a prospective cohort study in Germany, following children aged six to 16 years with at least one parent or sibling with T1D between 2009 and 2016 every six months up to the age of 18 (https://doi.org/10.1007/s00125-018-4688-x;PMID: 30008062).
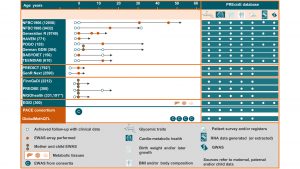
Preconceptual birth cohorts
- The Generation R Next is a prospective cohort study from preconception onwards (n=2,500) (www.generationr.nl/next); and
- The Rotterdam Periconceptional Cohort (Predict) is an ongoing cohort study (n=792 pregnancies) (https://academic.oup.com/ije/article/45/2/374/2572491).
Birth cohorts with dual EWAS: mother-child pairs
- The Finnish Gestational Diabetes Study (FinnGeDi) is the largest prospective cohort, comprising 1146 women and their offspring exposed to GDM and 1066 controls (mother-child pairs) (534 with EWAS data). Data were collected at seven delivery hospitals in Finland, between 2009 and 2012 (https://academic.oup.com/ije/article/49/3/762/5831097);
- The Role of Nutrition and Maternal Genetics on the Programming of Development of Foetal Adipose Tissue (PREOBE) study aims to obtain genetic and biochemical biomarkers for the programming of obesity in early stages of life (https://clinicaltrials.gov/ct2/show/NCT01634464);
- NIGOHealth investigates the effects of a low glycaemic index/slow digesting carbohydrates in obese pregnant mothers on their offspring health, with follow-ups being performed until the age of four years (https://clinicaltrials.gov/ct2/show/NCT02285764);
- PREcisE will also harness the data in the Biological Atlas from the European Genomic Institute for Diabetes (EGID) where genomics, epigenomics and transcriptomics have been performed (www.natureindex.com/institution-outputs/france/european-genomic-institute-for-diabetes-egid/5292c5c7140ba07c51000002/Life%20Sciences); and
- PREcisE is a member of the PACE and GlobalMeth eQTL consortia, enabling testing and replication of cord blood (5000 EWAS) and adult blood methylation signals (17000 EWAS).
Table 1 and 2 show availability of glycaemic, lifestyle, growth and sociodemographic data.
Following successful collaboration with the PACE consortium, the research community in epigenetic epidemiology has now gained a good general knowledge in the technical ability to perform epigenome-wide association studies. We are now able to reach out to an active network of longitudinal cohorts which provide a good statistical base to test the role of maternal glycaemic health and related nutritional factors on the child DNA methylation profile.
Challenge for large-scale epigenetic data on maternal and dietary exposure
GDM diagnosis criteria vary across the globe, as clinical perspectives on defining criteria for the cutoff values used differ between regions in spite of harmonisation efforts. The first large meta-analysis on association between GDM and the cord blood methylome uncovered that many birth cohorts do not have their glucose and insulin levels recorded, which hampered attempts to standardise the GDM diagnosis used in the meta-analysis3. Serum glucose, and to a lesser extent serum insulin, are measured and oral glucose tolerance tests (OGTT) performed in pregnancies worldwide; however, the stage of gestation at which these tests are undertaken varies widely, as does the testing scope – whether the whole population is screened or only at-risk pregnancies – and the manner of testing (fasted or non-fasted samples). To increase the available sample size for a meta-analysis on measurements of maternal gestational fasted glucose, insulin and OGTT on the neonatal epigenome, we made an international call through the PACE consortium for cohorts with these data – which are overall quite rare.
For this meta-analysis effort due to the complex relationships and networks between potentially contributing factors, we designed the analytical causal framework using directed acyclic graphs (DAG). This enabled us to select a minimal set of co-variables needed to assess a direct effect of the glycaemic variables (fasted glucose, insulin and OGTT) on the outcome. To obtain the DAG (Fig. 4) we referred to evidence of literature, including evidence from randomised placebo-controlled trials, MR studies, meta-analyses and other observational well-established links. Diet was flagged as one contributing factor in our DAG, but of course in reality it is a multifaceted issue.
In the combination of complex dietary scores with large scale meta-analyses of EWAS, we face a key challenge in the combination of different dietary assessment methods across human cohort studies. Food frequency questionnaires (FFQ), prospective dietary diaries and 24-hour dietary recalls are all often used, and all have their strengths and limitations20. FFQs, for example, have the advantages of time and cost effectiveness, user-friendly application, automated analysis, and coverage of the habitual diet. However, FFQs often miss data on specific food groups which are not frequently consumed, in particular if validation was not performed in the target population21. On the contrary, prospective dietary records and 24-hour recalls are more precise and more valid if performed over a longer period; but they lack information on a general diet (and rarely consumed food items) and are cost- and time-intensive22-23. Additionally, even if similar dietary assessment tools are applied, there can be substantial variation between studies in terms of factors such as the number of food items included in the FFQ. For example, FFQs can collect information about the consumption of from 50 to 300 distinct items, with varying level of detail per item; and FFQs can have differences in validity and reproducibility, which logically provides a different basis for dietary intake calculation. Thus, the heterogeneity arising from various methodological approaches for the assessment of dietary behaviour needs to be addressed.
As this consortium-based type of meta-analysis relies on previously collected data, we cannot change the design of individual studies; and therefore we have to optimise methods to harmonise the data from different sources and account for known factors influencing all types of dietary assessment methods, such as underreporting in overweight or obese people23. Hence, a meta-analysis of EWASs of dietary data is reasonable here, as all participating cohorts adhere to a predefined comprehensive analysis plan which specifies methods for dietary score calculation, statistical analyses and definition of covariates. Moreover, sensitivity analyses – for instance, only covering one specific dietary assessment method – will be performed to distinguish actual nutrition-related associations from bias based on methodological differences.
Data standards and where to access the data
One of the important scientific outputs of PREciSE in terms of data will be the epigenetic and other summary statistics of a series of epigenome-wide and candidate loci analyses that will be generated in concordance with practices pioneered by GWAS analyses. Such summary statistics offer a valuable resource for further scientific endeavours and will therefore be made publicly available, ensuring that they are findable, accessible, interoperable and reusable (FAIR), in PREciSE publications and added to relevant public repositories, such as dbGap or EWAScatalog and will be indexed with a DOI as a persistent and unique identifier.
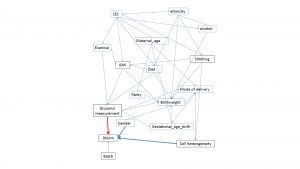
Additionally, newly generated data, such as the harmonised data, new variables and new statistical methodologies, will be documented and available. Metadata catalogues and scripts will be shared amongst the partners and disseminated publicly for third party access. For interoperability purposes, we will work together with related projects such as the JPI-funded projects ALPHABET and NutriPROGRAM and other Consortia such as the PACE consortium, to make sure we use existing standards where they exist and join hands to develop new standards where they do not exist.
Conclusion
The PREcisE project joins a consortium with complementary domains of expertise and data to uncover DNA methylation markers sensitive to variation by maternal glycaemic health in pregnancy, considering pre- and postnatal dietary exposures as possible modulators, and characterise their importance in explaining the mother-to-offspring transmission of impaired glycaemic health. We integrate human data spanning from pre-conception to age 50 years with dense DNA methylation data in the mother, the newborn and the adult with repeated measure to challenge the question of replicability, specificity and persistency. Effectively, the PREcisE consortium will organise its working plan into three work packages to i) perform epigenome wide association study on glucose level exposure in utero followed by downstream analysis in a biobank of liver, skeletal muscles and adipose tissues; ii) infer the primary role of pre- and postnatal dietary exposures in modulating the association of methylation with adiposity and glycaemic health outcomes; and iii) integrate its findings further into a life-course model for adiposity and glycaemic health outcomes trained in large birth cohort data.
Authors
Evangelia Tzala (1), Sylvain Sebert (7), Raffael Ott (4), Leanne K Küpers (3), Mercedes G Bermudez (5), Mickaël Canouil (2), Inga Prokopenko (1), Richard Saffery (6), Marja Vääräsmäki (7,8), Eero Kajantie (7,8), Cristina Campoy (5), Régine Steegers-Theunissen (3), Vincent W. Jaddoe (3), Phillippe Froguel (2), Priyanka Parmar (7), Amelie Bonnefond (2), Janine F. Felix (3), Sandra Hummel (4), Elmar W.Tobi (3), Marjo-Riitta Järvelin (1,7,8)
Acknowledgements
PREcisE is supported by the Joint Programming Initiative – A Healthy Diet for a Healthy Life (JPI HDHL) (proposal number 655). In the UK it is jointly funded by the Medical Research Council (MRC) and the Biotechnology and Biological Sciences Research Council (BBSRC) [grant reference: MR/S03658X/1]; in Spain by Instituto de Salud Carlos III [PCI2018-093147], in Germany by the German Federal Ministry of Education and Research [FKZ 01EA1905]; ZonMw in the Netherlands [529051023]; and in France by French National Research Agency [ANR-18-HDHL-0003-05].
References
1 Bjerregaard LG, Jensen BW, Ängquist L, Osler M, Sørensen TIA, Baker JL. Change in Overweight from Childhood to Early Adulthood and Risk of Type 2 Diabetes. N Engl J Med. 2018;378(14):1302-1312. doi:10.1056/NEJMoa1713231.
2 Vääräsmäki M, Pouta A, Elliot P, et al. Adolescent Manifestations of Metabolic Syndrome Among Children Born to Women With Gestational Diabetes in a General-Population Birth Cohort. Am J Epidemiol. 2009;169(10):1209-1215. doi:10.1093/aje/kwp020
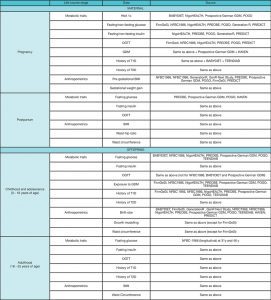
3 Howe C et al. Maternal Gestational Diabetes Mellitus and Newborn DNA Methylation: Findings From the Pregnancy and Childhood Epigenetics Consortium. Diabetes Care 2020 Jan;43(1):98-105. doi: 10.2337/dc19-0524. Epub 2019 Oct 10.
4 Tyrrell J, Richmond RC, Palmer TM, et al. Genetic Evidence for Causal Relationships Between Maternal Obesity-Related Traits and Birth Weight. JAMA. 2016;315(11):1129. doi:10.1001/jama.2016.1975.
5 Hedderson MM, Ferrara A, Sacks DA. Gestational diabetes mellitus and lesser degrees of pregnancy hyperglycaemia: association with increased risk of spontaneous preterm birth. Obstet Gynecol. 2003;102(4):850-856. www.ncbi.nlm.nih.gov/pubmed/14551018.
6 HAPO Study Co-operative Research Group, Metzger BE, Lowe LP, et al. Hyperglycaemia and adverse pregnancy outcomes. N Engl J Med. 2008;358(19):1991-2002. doi:10.1056/NEJMoa0707943.
7 Franks PW, Looker HC, Kobes S, et al. Gestational glucose tolerance and risk of type 2 diabetes in young Pima Indian offspring. Diabetes. 2006;55(2):460-465. www.ncbi.nlm.nih.gov/pubmed/16443781.
8 Hillier TA, Pedula KL, Schmidt MM, Mullen JA, Charles M-A, Pettitt DJ. Childhood obesity and metabolic imprinting: the ongoing effects of maternal hyperglycaemia. Diabetes Care. 2007;30(9):2287-2292. doi:10.2337/dc06-2361.
9 Zhang C, Rawal S, Chong YS. Risk factors for gestational diabetes: is prevention possible? Diabetologia. 2016;59(7):1385-1390. doi:10.1007/s00125-016-3979-3.
10 Viana LV, Gross JL, Azevedo MJ. Dietary intervention in patients with gestational diabetes mellitus: a systematic review and meta-analysis of randomised clinical trials on maternal and newborn outcomes. Diabetes Care. 2014;37(12):3345-3355. doi:10.2337/dc14-1530.
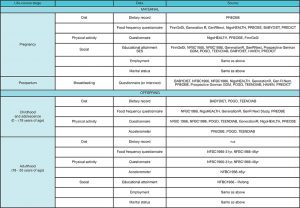
11 Barrès R, Zierath JR. The role of diet and exercise in the transgenerational epigenetic landscape of T2DM. Nat Rev Endocrinol. 2016;12(8):441-451. doi:10.1038/nrendo.2016.87.
12 Hartwig FP, Loret de Mola C, Davies NM, Victora CG, Relton CL. Breastfeeding effects on DNA methylation in the offspring: A systematic literature review. PLoS One. 2017;12(3):e0173070. doi:10.1371/journal.pone.0173070.
13 Obermann-Borst SA, Eilers PHC, Tobi EW, et al. Duration of breastfeeding and gender are associated with methylation of the LEPTIN gene in very young children. Pediatr Res. 2013;74(3):344-349. doi:10.1038/pr.2013.95.
14 Fraga MF, Ballestar E, Paz MF, et al. Epigenetic differences arise during the lifetime of monozygotic twins. Proc Natl Acad Sci USA. 2005;102(30):10604-10609. doi:10.1073/pnas.0500398102.
15 Kundaje A, Meuleman W, Ernst J, et al. Integrative analysis of 111 reference human epigenomes. Nature. 2015;518(7539):317-330. doi:10.1038/nature14248.
16 The ENCODE Project Consortium. An integrated encyclopedia of DNA elements in the human genome. Nature. 2012;489(7414):57-74. doi:10.1038/nature11247.
17 Chambers JC, Loh M, Lehne B, et al. Epigenome-wide association of DNA methylation markers in peripheral blood from Indian Asians and Europeans with incident type 2 diabetes: a nested case-control study. Lancet Diabetes Endocrinol. 2015;3(7):526-534. doi:10.1016/S2213-8587(15)00127-8.
18 Wahl S, Drong A, Lehne B, et al. Epigenome-wide association study of body mass index and the adverse outcomes of adiposity. Nature. 2017;541(7635):81-86. doi:10.1038/nature20784.
19 Vandenbroucke J, Broadbent A, Pearce N. Approaches to causal inference – causality and causal inference in epidemiology: the need for a pluralistic approach. Int Journ Epidem. 2016, 1776–1786doi: 10.1093/ije/dyv341
20 Shim J-S, Oh K, Kim HC. Dietary assessment methods in epidemiologic studies. Epidemiology and Health 2014;36:e2014009;
21 Cade JE, Burley VJ, Warm DL, Thompson RL, Margetts BM: Food-frequency questionnaires: a review of their design, validation and utilisation. Nutrition Research Reviews 2004 Jun;17(1):5-22. doi: 10.1079/NRR200370
22 Freudenheim J. A review of study designs and methods of dietary assessment in nutritional epidemiology of chronic disease. The Journal of Nutrition, Volume 123, Issue suppl_2, February 1993, Pages 401–405, https://doi.org/10.1093/jn/123.suppl_2.401, PMID: 8429394
23 Lichtman S et al. Discrepancy between Self-Reported and Actual Caloric Intake and Exercise in Obese Subjects. N Engl J Med 1992; 327:1893-1898. DOI: 10.1056/NEJM199212313272701
This article is from issue 18 of Health Europa. Click here to get your free subscription today.
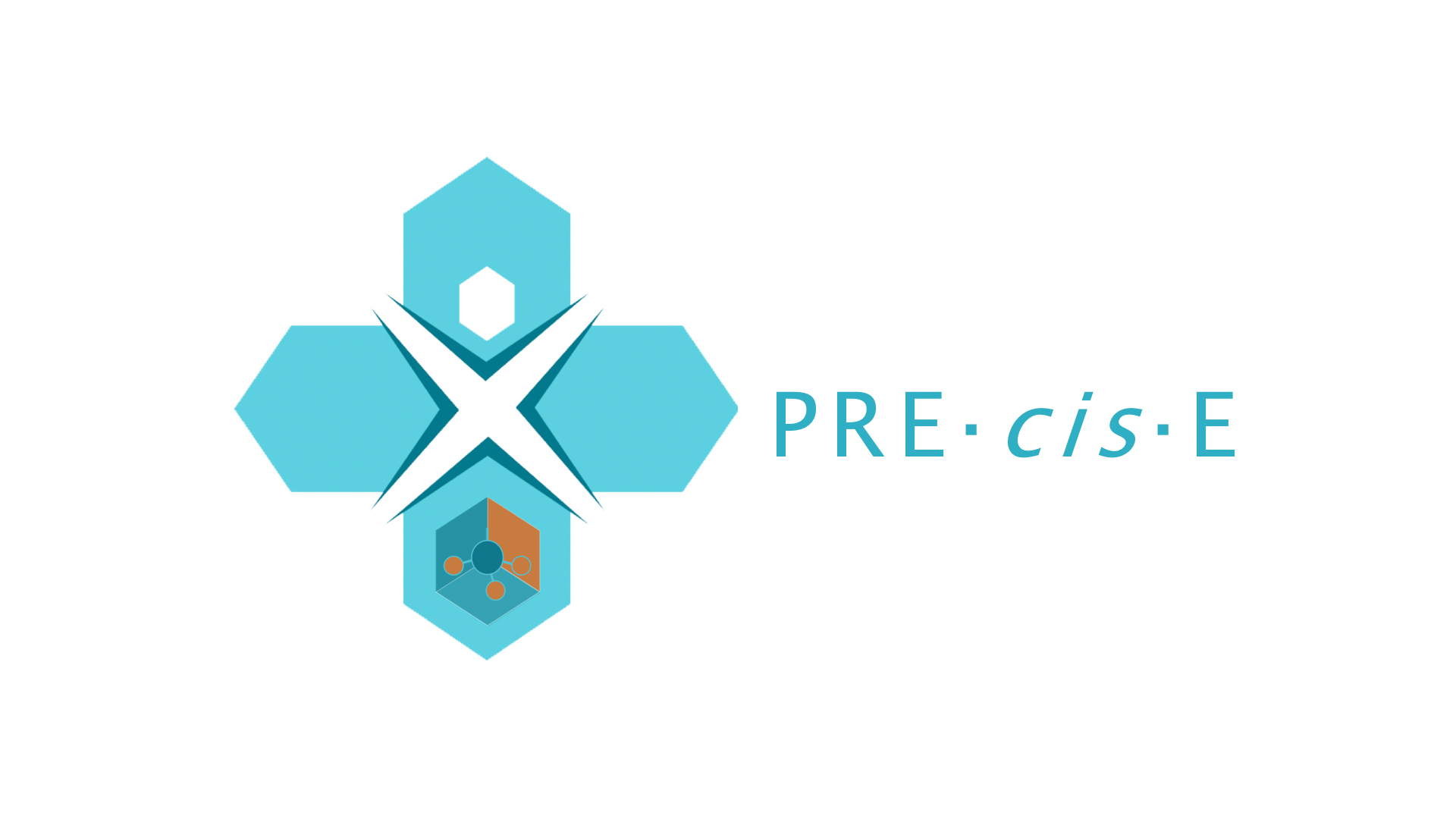