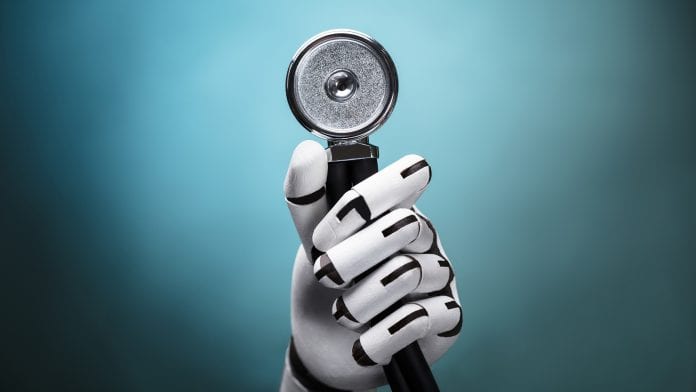
Artificial intelligence and machine learning (AI/ML) are increasingly transforming the healthcare sector.
Along with the benefits come new risks and regulatory challenges meaning doctors now have to consider how best to reduce risk when it comes to medical technology.
From spotting malignant tumours to reading CT scans and mammograms, Artificial Intelligence and Machine Learning-based technology is faster and more accurate than traditional devices – or even the best doctors.
In a new article, Algorithms on regulatory lockdown in medicine, recently published in Science, researchers look at the new challenges facing regulators as they navigate the unfamiliar pathways of Artificial Intelligence and Machine Learning.
Reducing risk in medical technology
In the paper, the researchers consider the questions: what new risks do we face as Artificial Intelligence and Machine Learning (AI/ML) devices are developed and implemented? How should they be managed? What factors do regulators need to focus on to ensure maximum value at minimal risk?
Until now regulatory bodies like the US Food and Drug Administration (FDA) have approved medical AI/ML-based software with ‘locked algorithms’, that is, algorithms that provide the same result each time and do not change with use.
However, a key strength and potential benefit from most AI/ML technology is derived from its ability to evolve as the model learns in response to new data. These ‘adaptive algorithms’, made possible because of AI/ML, create what is in essence a learning healthcare system, in which the boundaries between research and practice are porous.
Given the significant value of this adaptive system, a fundamental question for regulators today is whether authorisation should be limited to the version of technology that was submitted and evaluated as being safe and effective, or whether they permit the marketing of an algorithm where greater value is to be found in the technology’s ability to learn and adapt to new conditions.
The authors take an in-depth look at the risks associated with this update problem, considering the specific areas which require focus and ways in which the challenges could be addressed.
The key to strong regulation, they say, is to prioritise continuous risk monitoring.
The authors said: “To manage the risks, regulators should focus particularly on continuous monitoring and risk assessment, and less on planning for future algorithm changes.”
As regulators move forward, the authors recommend they develop new processes to continuously monitor, identify, and manage associated risks. They suggest key elements that could help with this, and which may in the future themselves be automated using AI/ML – possibly having AI/ML systems monitoring each other.
While the paper draws largely from the FDA’s experience in regulating biomedical technology, the lessons and examples have broad relevance as other countries consider how they shape their associated regulatory architecture.
They are also important and relevant for any business that develops AI/ML embedded products and services, from automotive, to insurance, financials, energy, and increasingly many others. Executives in all organisations have a lot to learn about managing new AI/ML risks from how regulators think about them today.
“Our goal is to emphasise the risks that can arise from unanticipated changes in how medical AI/ML systems react or adapt to their environments,” say the authors, warning that, “Subtle, often unrecognised parametric updates or new types of data can cause large and costly mistakes.”