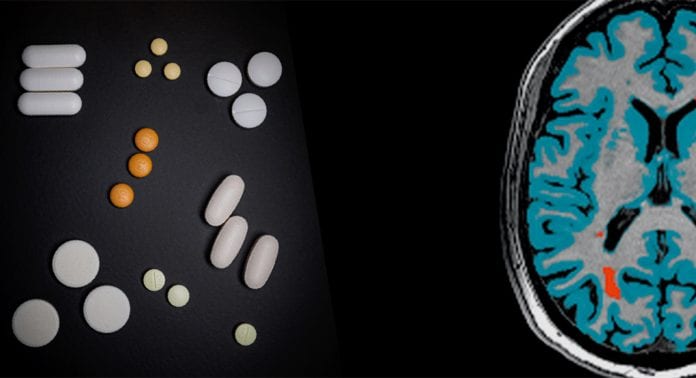
icometrix outlines the challenges of multiple sclerosis management and discusses personalised medicine solutions based on data-driven insights.
Every five minutes, someone, somewhere in the world is diagnosed with multiple sclerosis (MS)1. Today, more than 2.8 million people are living with MS, making it the most common progressive neurological condition in young people. The challenge in MS lies in its complex and chronic nature characterised either by periods of relapses and remission or a progressive disability pattern. Today, there are over 15 disease-modifying treatments available, aiming to slow down relapses and disease progression. Despite this pharmacological progress, the biggest challenge remains the matching of the right patient with the right drug at the right time.
One in four MS patients starts on a suboptimal treatment2. However, the health economic cost of this disconnect mainly results from the fact that these patients remain on such a suboptimal treatment for an average of four years. The solution to this mismatch is a tailor-made treatment strategy, enabled by personalised medicine to make healthcare smarter, better, and more cost-effective. Personalised treatment management in MS relies on three pillars: earlier detection of suboptimal treatment, better drug-matching of patients, and awareness and adoption of treatment paradigms. The sustainable implementation of these pillars requires data-driven, integrated, and scalable real-world solutions.
Early treatment escalation
The definition of treatment failure depends on the intention to suppress any clinical or subclinical disease activity, fully or partially. However, today, relapses are systematically underreported by half of MS patients, whereas disease progression often goes unnoticed during clinical assessments3,4. In addition, disease activity as assessed on magnetic resonance imaging (MRI), is often missed4. Hence, the ability to escalate treatments earlier, heavily relies on tools that are sensitive enough to detect disease activity and progression faster.
How can we achieve early escalation? The solution needs to be two-fold, capturing both clinical progression (symptoms, relapses, and disability), and the subclinical disease progression (lesion change and brain volume loss) observed in MS patients. Remote patient monitoring through medical health (mHealth) applications makes the continuous and more objective monitoring of symptoms possible. Regular standardised check-ins through validated mHealth apps can mitigate the underreporting of patients reported outcomes and bridge the information-gap between annual neurology visits. To ensure a closed information-loop, such mHealth apps need to be cleared for clinical use as a medical device. Also, they need to be integrated into a centralised dashboard for MS care teams, to allow MS care teams to be more efficient and better-informed.
MRI plays a central role in the monitoring of MS and detection of disease activity. However, MRI lesion change tracking in a clinical setting is tedious and is highly dependent on the experience and specialisation of radiologists. This prompted the introduction of assistive MRI software, which can increase the radiologist’s sensitivity and consistency in interpreting scans5,6. The inclusion of brain atrophy in treatment guidelines, initially qualitatively but recently also quantitatively, has made the inclusion of software-assisted brain volume loss computation essential4,7-10.
The introduction of such software-assisted MRI monitoring allows healthcare practitioners to confidently incorporate both lesion changes and brain atrophy into the clinical reporting and decision-making for MS patients. To maximise adoption and health outcomes, such solutions need to be validated, regulatory approved, globally available, and easily integrated into the patient’s health record.
Data-driven decision-making
Identifying the need to escalate or initiate a patient’s treatment gives rise to the more complex exercise of matching patients with the most suitable drug available. The transformation of the conventional disease-centred caregiving, (based on broad disease categories) towards personalised medicine, (based on individual characteristics, disease subtype, risk, prognosis, or treatment response) will help meet the unique needs of patients.
The key idea in personalised medicine is to base medical decisions on personal biomarkers, rather than on population averages. The source of such data can be medical imaging, health records, health claims, data from wearable sensors, and mHealth apps, next to genomics, metabolomics, etc. Such data-driven insights require the organisation and analysis of massive datasets to identify features that may indicate optimal treatment decisions. This is where machine learning (a subset of artificial intelligence, AI) can play an important role, by integrating multimodal data to generate actionable insights. The resulting predictive models can aid in improved patient stratification, prediction of progression, and therapeutic response11.
Personalised medicine has the advantage of reducing health costs by improving medication effectiveness, reducing adverse risks and side-effects by preventing unnecessary therapies, optimising the use of therapies, and improving disease-management by means of wearable technology and mHealth apps. Next to this, personalised medicine can contribute to the smarter design of clinical trials by selecting likely responders at baseline12,13.
The patient at the centre of the care pathway
Continuous innovation, pharmacological developments, and evolving treatment paradigms make the management of multiple sclerosis increasingly challenging. Healthcare professionals are extremely busy and can be easily overwhelmed by this fast-paced evolution. Tools that will help transform healthcare and make personalised medicine sustainable will therefore need an educational component to drive awareness and adoption for both caregiver and patient.
Digital therapeutics, wearable technology, and mHealth apps also play an essential role in the empowerment of patients by increasing awareness about their health and their ability to manage their own condition. Connecting caregivers and patients through such tools in an integrated and constructive manner will also ameliorate shared decision-making and can improve a patient’s commitment to therapy and their satisfaction, which in turn translates into better health outcomes.
Making it happen
The global and sustainable implementation of personalised medicine will depend on data-driven, integrated, validated, and scalable solutions. The transition from traditional to personalised care will be gradual and will evolve together with the development and adoption of software-assisted decision support tools, wearable technology, mHealth apps, and digital therapeutics. The key will be to integrate these solutions and capture, organise, analyse and interpret the resulting data to generate meaningful clinical insights.
Next to multiple sclerosis, personalised medicine also holds great promise in the management of other neurological conditions. Currently, around 50 million people are living with dementia, and it is expected that this number will rise to 150 million by 2050. The ongoing race to launch the first drug to slow down progression in Alzheimer’s disease will soon introduce therapeutic options into the management of dementia and with this the need to identify responders early in the disease progression.
icometrix strives to transform patient care through data-driven insights and personalised medicine, supported by Artificial Intelligence (AI). icometrix’s cloud-based AI solutions assist patients and healthcare practitioners in the management of neurological conditions and securely connect care teams. icometrix supports pharmaceutical companies in phase I-III and Real-World Evidence (RWE) studies through imaging and data services, and digital health strategy.
icobrain, an AI software solution that quantifies clinically meaningful changes on MRI and CT scans, is a CE-marked and FDA-cleared tool to help detect disease progression in individual patients and assist healthcare professionals in adopting treatment guidelines.
icompanion, an mHealth app for patients and integrated web platform for healthcare professionals, is a CE-marked remote monitoring solution that captures meaningful changes in clinical symptoms, cognition, disability, and fatigue.
icobridge and iconnect, powerful solutions that seamlessly integrate icobrain and icompanion in clinical trials and hospital workflows.
References
- Coetzee T, Thompson AJ. (2020). Atlas of MS 2020: Informing global policy change. Multiple Sclerosis Journal. 2020;26(14):1807-1808. doi:10.1177/1352458520968811
- Sa JS, et al. (2014). Relapsing–Remitting Multiple Sclerosis: Patterns of Response to Disease-Modifying Therapies and Associated Factors: A National Survey. Neurol Ther. 3(2): 89–99. doi: 10.1007/s40120-014-0019-4
- Duddy M, et al. (2014). The UK patient experience of relapse in Multiple Sclerosis treated with first disease modifying therapies. Mult Scler Relat Disord. 3(4):450-6. doi: 10.1016/j.msard.2014.02.006
- Giovannoni G, et al. (2016). Brain health: time matters in multiple sclerosis. Mult Scler Relat Disord. 9 Suppl 1:S5-S48. doi: 10.1016/j.msard.2016.07.003
- Dahan A, et al. (2018). Computer-Aided Detection Can Bridge the Skill Gap in Multiple Sclerosis Monitoring. Journal of the American College of Radiology: JACR, 15(1 Pt A), 93–96. doi: 10.1016/j.jacr.2017.06.030
- Sima DM, et al. On the use of icobrain’s prepopulated radiology reporting template for multiple sclerosis follow-up. ECR 2020 / C-11342
- Kappos L, et al. (2016). Inclusion of brain volume loss in a revised measure of ‘no evidence of disease activity’ (NEDA-4) in relapsing-remitting multiple sclerosis. Mult Scler. 22(10):1297-305. doi: 10.1177/1352458515616701.
- Montalban X, et al. (2018). ECTRIMS/EAN Guideline on the pharmacological treatment of people with multiple sclerosis. Mult Scler. 24(2):96-120. doi: 10.1177/1352458517751049.
- Sastre-Garriga J, et al. (2020). MAGNIMS consensus recommendations on the use of brain and spinal cord atrophy measures in clinical practice. Nat Rev Neurol. (3):171-182. doi: 10.1038/s41582-020-0314-x.
- Pérez-Miralles FC, et al. (2020) Adding brain volume measures into response criteria in multiple sclerosis: the Río-4 score. Neuroradiology. doi: 10.1007/s00234-020-02604-8.
- Fröhlich H, et al. (2018). From hype to reality: data science enabling personalized medicine. BMC Med 16, 150. doi: 10.1186/s12916-018-1122-7.
- Mathur S, Sutton J. (2017). Personalized medicine could transform healthcare. Biomed Rep. 7(1):3-5. doi: 10.3892/br.2017.922.
- Schork NJ. (2019). Artificial Intelligence and Personalized Medicine. Cancer Treat Res. 178:265-283. doi:10.1007/978-3-030-16391-4_11