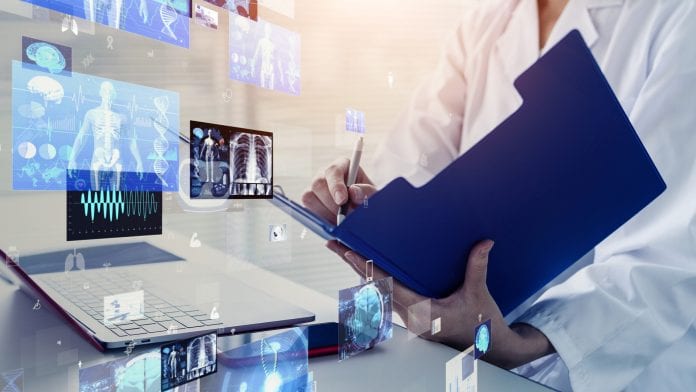
How smart monitoring technologies and AI help healthcare professionals predict adverse outcomes before they happen in the perioperative care pathway.
In Europe, 2.4 million patients undergo high-risk surgery every year and one out of four develop serious complications post-surgery1,2. Fortunately, death during surgery is now rare. Yet, postoperative complications leading to death after surgery remain considerable with more than one in 100 adults (1.5%) dying within the first 30 days post-procedure1.
Throughout the hospital patient journey, anaesthetists and intensivists need to analyse large amounts of data to make life-critical decisions for patients. One example is blood pressure changes known as haemodynamic instability. Drops in blood pressure during surgery, or intraoperative hypotension (IOH), are a common side effect of general anaesthesia and can have a significant impact on patient safety after surgery. Even short periods of IOH are linked with severe postoperative events such as myocardial or acute kidney injury3. These complications come with a significant burden for healthcare systems as they are strongly linked with mortality, morbidity, and rehospitalisation4.
Patient monitoring systems have been used in operating rooms (ORs) and intensive care units (ICUs) to monitor blood pressure and other vital signs for decades4,5. However, with these technologies, IOH is often not detected and when it is the treatment is reactive, occurring after the blood pressure has already dropped below safe levels6. More recently, advanced predictive monitoring is being used to anticipate and preventively manage IOH. This type of smart technology uses Big Data to predict clinical changes and has demonstrated success in predicting and therefore reducing IOH7,8. However, in Europe today it is estimated that only about 10% of high-risk surgeries are monitored using advanced haemodynamic monitoring, and fewer than 1% of those patients are benefiting from the latest smart monitoring technologies1,2.
Hypotension prediction using AI
Edwards Lifesciences has developed the Acumen Hypotension Prediction Index (HPI)™9 software which provides clinicians with information regarding the likelihood that a patient will suffer from IOH. It has been developed together with healthcare professionals across the world and leverages predictive analytics to alert anaesthetists in the OR and intensivists in the ICU of potential blood pressure drops before they occur.
Edwards Lifesciences’ Vice President for Critical Care and Vascular Europe, Carine Schorochoff, believes that digital health technologies have the potential to transform patient monitoring in the emergency room (ER), as well as in the OR and the ICU, whilst improving quality of care and increasing patient safety. This will contribute to creating efficiencies in hospital settings and strengthening resilience of healthcare systems, especially post-COVID-19.
Why is AI increasingly important for patient safety?
AI technologies allow clinicians to anticipate patient failure, providing them time to take preventative measures. At Edwards Lifesciences, patient safety is at the core of all we do. We utilise Big Data and machine learning to develop breakthrough patient monitoring solutions. We develop predictive algorithms, which anticipate the risk of patient failure. This ability to anticipate augments the role of clinicians by allowing them to take preventive actions, therefore reducing the risk of complications. AI provides critical insights to guide proactive treatment decisions for patients undergoing high-risk surgery or patients who are critically ill admitted to the ICU.
What are the benefits of predictive monitoring?
Predictive monitoring generates several benefits for patients and healthcare systems.
First, it allows the detection of rapid physiologic changes – in this case, a critical drop in blood pressure – in critically ill patients ahead of time, both in ORs and ICUs. By preventing adverse events, it contributes to a reduction in the risks of patient failure, thereby reducing the risk of serious complications. Already today, data suggests that predictive applications have the potential to help save thousands of lives yearly10.
Second, these solutions can support healthcare systems in setting a safer patient pathway through the hospital, in the ER, the OR and the ICU. Such an integrated process will enhance patient safety by reducing adverse events during high-risk surgery as well as during recovery in the ICU11, thereby improving patient outcomes. Furthermore, AI-based technologies will also provide access to multiple specific patients’ parameters, allowing a more personalised treatment.
Finally, digital AI-based technologies can deliver resource savings12 by lowering complications and reducing the length of a patient’s stay in hospital. These results will allow for the hospital workforce allocation to be optimised, especially in the ICU, where healthcare systems are currently experiencing staff shortages and are under huge pressure.
Which types of patients can predictive monitoring help?
Predictive monitoring is usually needed for patients undergoing high-risk surgery or patients who are critically ill and admitted to the ICU. It will also provide great support in the ER, allowing clinicians to better identify the patients who require immediate attention.
What is your vision for the future of digital health technologies?
We will definitely see an increase in the adoption of digital health technologies.
The COVID-19 pandemic has drastically accelerated the need for solutions that will improve patient safety throughout the hospital journey. These new technologies will support clinicians in the monitoring of critically ill patients, helping to improve the quality of care, therefore reducing complications and length of stays. This will alleviate the pressure on the overall healthcare system.
At Edwards Lifesciences, we are committed to partnering with clinicians to improve patient safety. We invest substantially in research and development and along with predictive monitoring algorithms, such as HPI, we are developing prescriptive algorithms aimed at supporting clinicians in their treatment strategies. We are also working on solutions to provide clinicians with patient parameters in real time on mobile devices. Consequently, clinicians will be able to monitor ICU patients remotely, improve the quality of care and, again, reduce the burden on healthcare systems.
References
1 Ghaferi AA et al. Variation in hospital mortality associated with inpatient surgery. N Engl J Med, 2009.
2 Preoperative Score to Predict Postoperative Mortality (POSPOM). Edward’s presentation.
3 Walsh M et al. (2013). Relationship between intraoperative mean arterial pressure and clinical outcomes after noncardiac surgery: toward an empirical definition of hypotension. Anesthesiology 119(3), 507-515.
4 Michard F, Biais M, Lobo SM, Futier E. Perioperative haemodynamic management 4.0. Best Pract Res Clin Anaesthesiol. 2019 Jun;33(2):247-255. Available at https://pubmed.ncbi.nlm.nih.gov/31582103/ [accessed March 2021].
5 Michard F. Decision support for haemodynamic management: from graphical displays to closed loop systems. Anesth Analg. 2013 Oct;117(4):876-82. Available at https://journals.lww.com/anesthesia-analgesia/Fulltext/2013/10000/Decision_Support_for_Hemodynamic_Management__From.16.aspx [accessed March 2021].
6 Wijnberge M et al. The use of a machine learning algorithm that predicts hypotension during surgery in combination with personalised treatment guidance: study protocol for a randomised clinical trial. Trials. 2019 Oct 11;20(1):582.
7 Rothstein WB, Navarrete SB, Scott MJ. Predictive haemodynamic monitoring in cardiac surgery: an observational validation study of the Hypotension Prediction Index. Cadiorthorac Surg. 2019 Oct; 229(4):s48. Available at www.journalacs.org/article/S1072-7515(19)30575-7/fulltext [accessed March 2021].
8 Schneck E et al. Hypotension Prediction Index-based protocolised haemodynamic management reduces the incidence and duration of intraoperative hypotension in primary total hip arthroplasty: a single centre feasibility randomised blinded prospective interventional trial. J Clin Monit Comput. 2020 Dec;34(6):1149-1158. Available at https://pubmed.ncbi.nlm.nih.gov/31784852/ [accessed March 2021].
9 The Acumen HPI software received de novo clearance from the US Food and Drug Administration in March 2018 and the CE mark in October 2016.
10 MedTech Europe. The socioeconomic impact of AI in healthcare. www.medtecheurope.org/resource-library/the-socio-economic-impact-ofai-in-healthcare-addressing-barriers-to-adoption-for-new-healthcare-technologies-in-europe/
11 Rotter T et al. Clinical pathways: effects on professional practice, patient outcomes, length of stay and hospital costs. Cochrane Database Syst Rev. 2010;(3):CD006632. Published 2010 Mar 17. doi:10.1002/14651858.CD006632.pub2
12 Schrijvers G et al. The care pathway: concepts and theories: an introduction. International journal of integrated care vol. 12,Spec Ed Integrated Care Pathways e192. 18 Sep. 2012, doi:10.5334/ijic.812
For professional use. See instructions for use for full prescribing information. CE marked medical device.
© 2021 Edwards Lifesciences Corporation. All rights reserved. PP–EU-1914 v1.0
Edwards Lifesciences · Route de l’Etraz 70, 1260 Nyon, Switzerland · edwards.com
Edwards Lifesciences
www.edwards.com
This article is from issue 17 of Health Europa. Click here to get your free subscription today.