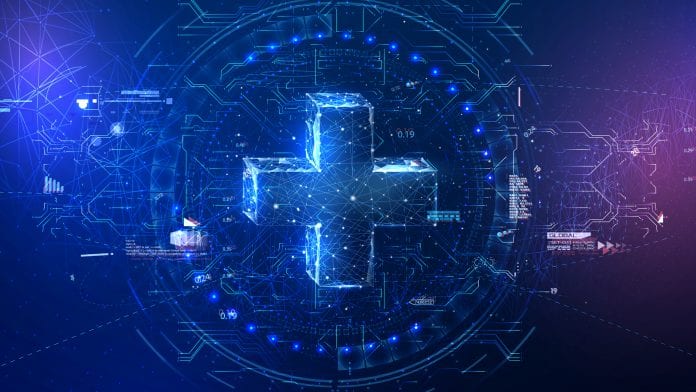
A new, experimental Artificial Intelligence tool is helping in the fight against COVID-19 by predicting which patients may develop respiratory disease from the virus.
The Artificial Intelligence (AI) tool has been shown to accurately predict which patients that have been newly infected with the COVID-19 virus would go on to develop severe respiratory disease.
Named SARS-CoV-2, the new novel coronavirus, as of March 30, had infected 735,560 patients worldwide. According to the World Health Organization, the illness has caused more than 34,830 deaths to date, more often among older patients with underlying health conditions.
The study, published in the journal Computers, Materials & Continua, was led by NYU Grossman School of Medicine and the Courant Institute of Mathematical Sciences at New York University, in partnership with Wenzhou Central Hospital and Cangnan People’s Hospital, both in Wenzhou, China.
Identifying vulnerable patients with AI
The study has revealed the best indicators of future severity and found that they were not as expected.
Corresponding author Megan Coffee, clinical assistant professor in the Division of Infectious Disease & Immunology at NYU Grossman School of Medicine, said: “While work remains to further validate our model, it holds promise as another tool to predict the patients most vulnerable to the virus, but only in support of physicians’ hard-won clinical experience in treating viral infections.”
“Our goal was to design and deploy a decision-support tool using AI capabilities – mostly predictive analytics – to flag future clinical coronavirus severity,” says co-author Anasse Bari, PhD, a clinical assistant professor in Computer Science at the Courant institute. “We hope that the tool, when fully developed, will be useful to physicians as they assess which moderately ill patients really need beds, and who can safely go home, with hospital resources stretched thin.”
Unexpected predictors
For the study, demographic, laboratory, and radiological findings were collected from 53 patients as each tested positive in January 2020 for COVID-19 at the two Chinese hospitals. In a minority of patients, severe symptoms developed with a week, including pneumonia.
The researchers wanted to find out whether AI techniques could help to accurately predict which patients with the virus would go on to develop Acute Respiratory Distress Syndrome or ARDS, the fluid build-up in the lungs that can be fatal in the elderly.
To do this they designed computer models that make decisions based on the data fed into them, with programmes getting “smarter” the more data they consider. Specifically, the current study used decision trees that track series of decisions between options, and that model the potential consequences of choices at each step in a pathway.
The AI tool found that changes in three features – levels of the liver enzyme alanine aminotransferase (ALT), reported myalgia, and haemoglobin levels – were most accurately predictive of subsequent, severe disease. Together with other factors, the team reported being able to predict risk of ARDS with up to 80% accuracy.
ALT levels, which rise dramatically as diseases like hepatitis damage the – liver, were only a bit higher in patients with COVID-19, but still featured prominently in prediction of severity. In addition, deep muscle aches (myalgia) were also more commonplace and have been linked by past research to higher general inflammation in the body.
Lastly, higher levels of haemoglobin, the iron-containing protein that enables blood cells to carry oxygen to bodily tissues, were also linked to later respiratory distress. Could this be explained by other factors, like unreported smoking of tobacco, which has long been linked to increased haemoglobin levels?
Of the 33 patients at Wenzhou Central Hospital interviewed on smoking status, the two who reported having smoked, also reported that they had quit.
Limitations of the study, say the authors, included the relatively small data set and the limited clinical severity of disease in the population studied.
“I will be paying more attention in my clinical practice to our data points, watching patients closer if they for instance complain of severe myalgia,” adds Coffee. “It’s exciting to be able to share data with the field in real time when it can be useful. In all past epidemics, journal papers only published well after the infections had waned.”