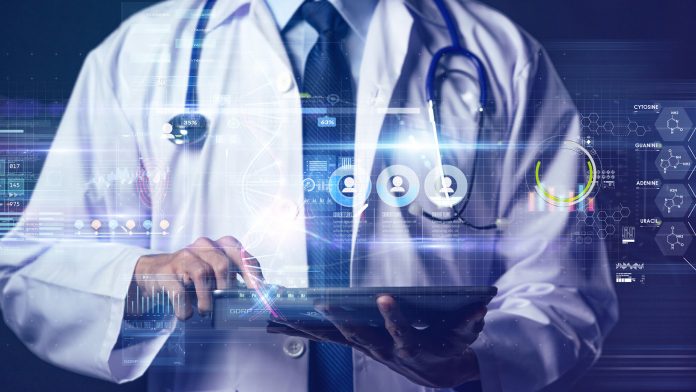
Researchers have developed an innovative Artificial Intelligence (AI) system that expertly identifies early signs of Type 2 diabetes.
Innovated by a team of researchers at the National Institutes of Health Clinical Center, the fully-automated, AI deep learning model exhibited excellent performance at locating early signs of Type 2 diabetes on abdominal CT scans – the current method for diagnosing the disease that is currently time-consuming to perform manually.
The study’s findings are published in the journal Radiology.
Importance of early diagnosis
Current data shows that around 13% of all adults in the US are affected by Type 2 diabetes, with a further 34.5% meeting the criteria for prediabetes. The signs of Type 2 diabetes can be slow to present themselves, meaning it is crucial to diagnose the disease in its early stages. For example, prediabetes can last up to eight years, and an early diagnosis can enable patients to make lifestyle changes that can mitigate the progression of the disease.
Limitations of current methods
Abdominal CT imaging has emerged as an effective method for diagnosing Type 2 diabetes and can illustrate extensive information about the pancreas. Earlier research found that patients with diabetes are likely to accumulate more visceral fat and fat within the pancreas than people without the disease. However, the liver, muscles, and blood vessels around the pancreas are relatively unstudied.
Hima Tallam, the first author of the study, commented: “The analysis of both pancreatic and extra-pancreatic features is a novel approach and has not been shown in previous work to our knowledge.”
One of the drawbacks of analysing low-dose non-contrast pancreatic CT images is that they have to be done manually by a radiologist or trained specialist, creating a time-consuming and difficult process. To address this, the team endeavoured to design an automated system to examine the pancreas.
Employing AI to detect signs of Type 2 diabetes
To perform their research, the team used a dataset of 8,992 patients who had been screened for colorectal cancer with CT between 2004 and 2016 at the University of Wisconsin Hospital and Clinics. 572 of the individuals had been diagnosed with Type 2 diabetes and 1,880 with dysglycemia, a condition in which blood sugar levels are high or too low. There was no overlap between diabetes and dysglycemic diagnosis.
The researchers developed their deep learning method using 471 images acquired from a range of datasets, including the Medical Data Decathlon, The Cancer Imaging Archive and the Beyond Cranial Vault challenge. Subsequently, the datasets were divided into three subsets, 424 for training, eight for validation, and 39 for test sets. Additionally, the team included data from four rounds of active learning.
The novel AI method achieved exceptional results, demonstrating virtually no difference to the manual analysis. Beyond its array of pancreatic features, the model also analysed the visceral fat, density and volumes of the surrounding abdominal muscles and organs, with results showing that diabetes patients had lower pancreas density and higher visceral fat than non-diabetes patients.
Doctor Ronald M. Summers, MD, PhD, the co-senior author of the study and senior investigator and staff radiologist at the National Institutes of Health Clinical Center, commented: “We found that diabetes was associated with the amount of fat within the pancreas and inside the patients’ abdomens. The more fat in those two locations, the more likely the patients were to have diabetes for a longer period of time.”
The most effective predictors of early signs of Type 2 diabetes in the final model included intrapancreatic fat percentage, pancreas fractal dimension, plaque severity between the L1-L4 vertebra level, average liver CT attenuation, and BMI, with the model using these predictors to distinguish between patients with and without diabetes accurately.
The researchers concluded: “This study is a step towards the wider use of automated methods to address clinical challenges. It may also inform future work investigating the reason for pancreatic changes that occur in patients with diabetes.”