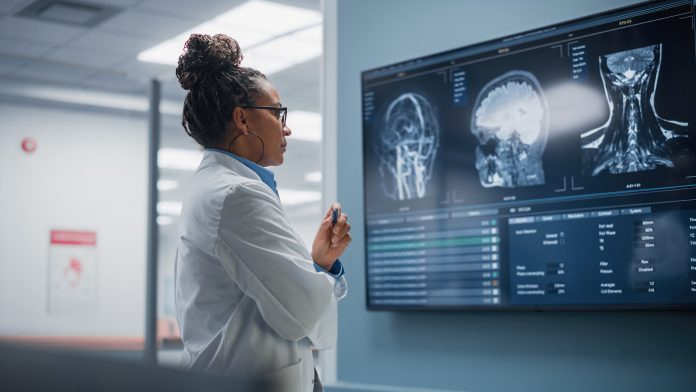
An innovation by Georgia State University in Artificial Intelligence could capture novel brain patterns associated with mental illness.
New research by Georgia State University’s TReNDS Center could revolutionise the early diagnosis of various mental illnesses by employing Artificial Intelligence (AI) and brain imaging. A team of scientists designed a computer programme that analysed brain imaging data to reveal patterns linked to mental illness conditions.
The development will support the diagnosis of mental illnesses, giving sufficient time to help prevent and treat these disorders more easily.
The study was published in Scientific Reports.
Employing functional magnetic resonance imaging (fMRI) to recognise mental illness
The scientists utilised fMRI to supply data to their computer programme, which measures dynamic brain activity by detecting tiny changes in blood flow.
“We built Artificial Intelligence models to interpret the large amounts of information from fMRI,” said Sergey Plis, Associate Professor of Computer Science and Neuroscience at Georgia State, and lead author of the study.
Plis compared fMRI imaging to a movie- he noted that: “The available data is so much larger, so much richer than a blood test or a regular MRI. But that’s the challenge—that huge amount of data is hard to interpret.”
Moreover, using fMRIs to spot mental illness indicators can be expensive and difficult to source. When combining fMRI with an AI model, it supports data mining meaning this data is available in large quantities.
“There are large datasets available in individuals without a known clinical disorder,” explained Vince Calhoun, Founding Director of the TReNDS Center and one of the study’s authors. Using these large but unrelated available datasets improved the model’s performance on smaller specific datasets.
“New patterns emerged that we could definitively link to each of the three brain disorders,” Calhoun said.
Training the AI model on over 10,000 scans
In the study, the scientists trained the AI model on a dataset of over 10,000 scans to understand basic fMRI imaging and brain function. Following this, the scientists employed multi-site data sets of over 1,200 individuals with mental illness diagnoses such as autism and schizophrenia.
The AI model began to learn current behaviour to accurately predict future behaviour. The software could focus on the ‘moment’ when the imaging data found the indicator of the mental illness in question.
To make the findings clinically useful, they will need to be applied before the onset of a mental illness. “If we can find markers for and predict Alzheimer’s risk in a 40-year-old,” Calhoun said, “we might be able to do something about it.”
“Even if we know from other testing or family history that someone is at risk of a disorder such as Alzheimer’s, we are still unable to predict when exactly it will occur,” Calhoun said. “Brain imaging could narrow down that time window by catching the relevant patterns when they do show up before clinical disease is apparent.”
“The vision is that we collect a large imaging dataset, our AI models pore over it, and show us what they learned about certain disorders,” Plis said. “We are building systems to discover new knowledge we could not discover on our own.”
“Our goal,” said Md Mahfuzur Rahman, first author of the study and a doctoral student in Computer Science at Georgia State, “is to bridge big worlds and big datasets with small worlds and disease-specific datasets and move towards markers relevant for clinical decisions.”