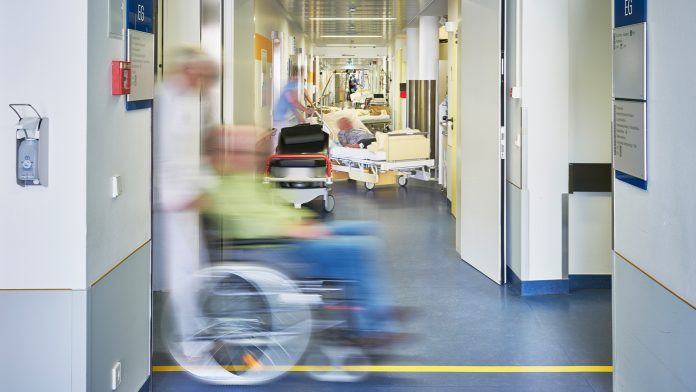
Unscheduled Care (UC) services provide substantial health benefits, but increasing demand is leading to unsustainable pressure on these services – discover the CUREd dataset, an urgent and emergency care dataset that provides enormous opportunities to provide maximum patient and health service benefit.
A little insight into the world of unscheduled care
Unscheduled Care (UC) services ) provide substantial health benefits for the population, but increasing demand is leading to unsustainable pressure on these services. Care is provided within this system through contact with a number of services and healthcare professionals – for example, calling an ambulance can lead to contact with paramedics who then transport the patient to the emergency department, where assessment and care by different professionals happens. Understanding how the public access and use unscheduled care is key to delivering improvements in services and patient outcomes.
However, care is provided within a system and therefore the ability to map pathways from call to discharge is essential. The CUREd dataset is an unscheduled care dataset that provides enormous opportunities to provide maximum patient and health service benefit. It was developed to link patient care across the whole unscheduled care system, allowing patient journeys to be followed.
An overview of the current unscheduled care system
In the English National Health Service (NHS) in 2012-13 there were 18.3 million attendances at major Emergency Departments (ED), single specialty EDs, walk-in centres and minor injury units, at a cost of £2.1 billion (~€2.35 billion). There were 5.3 million emergency hospital admissions, at a cost of £12.5 billion, 7 million ambulance service journeys and approximately 24 million calls to NHS unscheduled care telephone services.
Failure of the to manage increasing demand causes substantial public concern and political impact. Moreover, delays in ambulance response or Emergency Department (ED) assessment leads to worse patient outcomes. ED crowding is internationally recognised and is shown to be associated with avoidable mortality.
Of particular interest are the most vulnerable groups within the population who typically tend to be more frequent users of unscheduled care due to living with multiple long-term conditions and the consequences of ageing. These patients often have healthcare needs which have not been met resulting in them tipping into unscheduled care for their routine as well as urgent healthcare needs, or entering the revolving door of hospital admission – readmission.
Many patients are extremely complex and not only have physical health, but mental health needs and social care requirements. They are often the hardest to reach patient groups for participation in clinical trials, and as such developing cutting-edge solutions to their healthcare needs is not prioritised.
Making datasets available
The CUREd (unscheduled care system) dataset covers a population of 5.5 million (Yorkshire & Humber region, UK) from 2011, and links all patient episode data from 999/111 call to emergency department attendance and acute hospital admission and readmission (14 acute trusts) for adults and children.
There are over 15 million episodes of care in the dataset. Approvals for the dataset were obtained through NHS HRA and section 251 CAG approval, such that data is fully identifiable for linkage purposes and subsequently de-identified for research. Patient inclusion is on an opt-out basis. Approval for the dataset by the HRA as a research database means the data is ready for research, available for multiple use purposes, and wider linkage with related datasets.
Examples of further linkage currently being undertaken include linking mental health trust data and also hospice data (for studying end of life care). Opportunities exist for substantial expansion of this linked dataset into other geographical areas of the North through opportunities through Health Data Research UK (HDRUK).
The enormous opportunity of a linked dataset
The importance of a linked dataset describing a system of care provides enormous opportunities to follow and describe pathways of care for patients accessing the system. This approach is very patient-focused, but also provides an overview of where interventions should be targeted in order to provide maximum patient and health service benefit. The following are examples of how the dataset has been used to understand patient pathways and outcomes:
- Linkage between NHS111 call data and ED attendance. This was the first ever study to link these datasets demonstrated the proportion of patients being advised to attend ED vs. those who actually attended ED and their subsequent outcomes. The study showed that 23% of NHS111 calls go to ED as well, and 50% are advised not to attend. Accuracy of triaging calls is poor and need for ‘smart’ strategies for supporting decision making for call handlers is evident;
- Algorithmic approaches to identifying patients with an avoidable ED attendance. The dataset identified 23% adults and 30% children attend ED avoidably with a problem that could be managed in lower level of care (e.g. GP, pharmacy, self-care). This demonstrates where ‘smart’ triage could be used to reduce demand within the system if supported by interventions to facilitate patient-decision making;
- Algorithms applied to ambulance service data demonstrated 1:6 ambulance journeys transport patients to ED avoidably. Interventions could develop ‘smart’ tools for early identification and disposition decisions to be made by paramedics. According to the study, there is work commencing in 2019 on this; and
- Understanding geographical variation between hospitals in acute hospital admission for older patients (rates vary 43-70%), especially with short stays (rates vary 23-48%) who would benefit from interventions to keep them at home, or access same day emergency care.
Future key areas of work
These proposed areas of work can start to be delivered with existing data, but as data curation improves and the dataset becomes more detailed there will be the ability to deliver more accurate decision support to clinicians, services and patients.
Reducing avoidable ambulance journeys: utilising data-driven solutions to improve accuracy of call (999/111) disposition for ambulance and 111 services to ensure the right response attends the right patient. Leading to improvements in response times, demand management (using a menu of different responses from telephone to face to face), safely reducing avoidable ambulance transports.
Improving on-scene decision support for ambulance crews. Providing targeted decision support tools for paramedics to treat and leave on scene using linked data to drive algorithms.
Avoidable hospital admissions and readmissions: machine learning approaches that identify early avoidable hospital admissions thereby improving patient assessment and triage, decision making in ED and resource use within acute hospitals. It is important that such interventions also address inequalities through targeting vulnerable patient groups including the elderly, those with mental health problems, multi-morbidity, high users of services and patients eligible for same day emergency care.
Reducing low acuity attendances within the UEC system: improve and refine existing algorithmic approaches to identification of avoidable ED attendances. This would facilitate the development and testing of digital technological solutions aimed at directing low acuity ED attendances to a more appropriate level of care
References
- House of Commons Health Committee. Winter Pressure in Accident and Emergency Departments Third Report of Session 2016-17 Report, Together with Formal Minutes Relating to the Report.; 2016. www.parliament.uk.
- Crilly J, Keijzers G, Tippett V, et al. Improved outcomes for emergency department patients whose ambulance off-stretcher time is not delayed. Emerg Med Australas. 2015;27(3):216-224. doi:10.1111/1742-6723.12399
- Guttmann A, Schull MJ, Vermeulen MJ, Stukel TA. Association between waiting times and short term mortality and hospital admission after departure from emergency department: population based cohort study from Ontario, Canada. BMJ. 2011;342:d2983. doi:10.1136/bmj.d2983
Over There are over 15 million episodes of care in the dataset!!
amazing