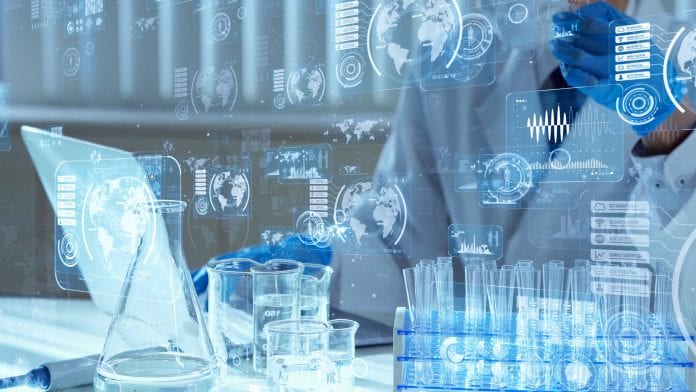
Patrick Malléa and Jérémy Clech of the AI4EU consortium tell HEQ about the benefits and challenges offered by AI in clinical research and care.
Healthcare delivery and research is increasingly integrating Artificial Intelligence (AI) technologies as a means of generating and assessing patient data. Patrick Malléa and Jérémy Clech of the AI4EU consortium tell HEQ about the benefits and challenges offered by AI in the clinical research and care sectors.
How could wider adoption of Artificial Intelligence (AI) technologies improve healthcare delivery? Is AI likely to play a larger role in healthcare in the future?
Patrick Malléa: Providing care is just one step within the much larger context of the health course. In terms of care, the health course includes an upstream phase dedicated to the prevention or detection of a disease, and a downstream phase dedicated to the management of the disease (access and co-ordination of care, avoiding aggravation of symptoms). The challenge in health, more than ever, is not limited to providing care, but rather to bring to the patients the best possible quality of life with their chronic or long-term disease. Therefore the health course is extended to include patients’ return home and remote monitoring. By intervening in each stage, by providing a personalised response adapted to each person which can be made available close to where they live, and by managing this health course as a whole, we can improve the quality of life of our fellow citizens. AI can help in all of those situations.
Caregivers do a remarkable job every day, and it is important that our health systems remain anthropocentric so that the human relationship continues to be at the heart of the care. In this way, the contribution of AI must enable patients and caregivers to have additional means to improve the quality of the medical service provided, to tend towards optimal organisations (availability, permanence and proximity) and to strengthen their expertise and decision-making capacity in order to provide the best care.
Could AI and machine learning be deployed to optimise healthcare provision within the context of COVID-19, for example by predicting patient needs and identifying at-risk groups?
Jérémy Clech: It should be noted that the issue of obtaining strictly legal access to enough high-quality data makes the realisation of AI programs very difficult, whether in France, Europe or across the world. The challenge today is no longer to produce algorithms, but to do it in a short time: from data collection until the deployment of the solution in production.
In the context of COVID-19, we have to deal with health data; and this is not an easy task. Obviously, it is necessary to be General Data Protection Regulation (GDPR)-compliant, but any research protocol using patient data also has to be approved by the authorities of the country in which it takes place.
An alternative is to collect anonymised data, even if this operation is far from easy. The healthcare facilities must then be mobilised to get patients’ consent to anonymise and transfer the data. In addition, the facilities’ staff, already under intense pressure, have to carry out these operations. Don’t think the job is done once the AI solution is produced and evaluated: it must then be integrated into the healthcare environment, in order to feed the AI algorithms with patients’ data and integrate AI results into the work process.
For several years our company, NEHS Digital, has been working to industrialise those steps as much as possible in the world of healthcare; in particular within the context of medical images. In association with the French institutions SFR and CERF, we organised the collection of anonymised data from chest CT scans of patients with suspected COVID-191. In collaboration with AI4EU and radiologists participating in scientific boards, the project launched in March 2020; and data collection, using our health data hosting infrastructure, began in May. An AI project aimed at predicting the probability of COVID-19 using CT scans was initiated in June and data scientists began work on the dataset in August. In December 2020 we deployed a first version in a pre-production environment. This was done in nine months. In summation, it is possible to deliver good results; but some significant efforts have to be made to get there.
What key features are necessary to ensure AI programs are human-centred? Why is this important?
PM: For me, technological progress and its acceleration in the 21st century cannot be conceived without an ethical approach. As such, the work undertaken by the European Commission in the context of ensuring the trustworthiness of AI constitutes good ethical practice and must govern any AI approach. These overarching principles are:
- Respect for human autonomy
- Prevention of harm
- Fairness
- Explicability
Taking these governing principles into account, seven key requirements for any AI initiative can be identified:
- Human agency and oversight
- Technical robustness and safety
- Transparency
- Diversity
- Non-discrimination and fairness
- Societal and environmental wellbeing
- Accountability
AI is a set of methods and tools; and it is only the ways in which it is used which define a positive or dangerous behaviour. We have seen through the initial feedback that the desired results can be achieved. In healthcare, AI works to strengthen the capacity of the caregiver and provides a framework for data gathering and analysis – this framework should allow data scientists and software engineers to handle any outstanding issues, ideally during the design phase, or at least before bringing an AI product to the market.
Particularly in the context of healthcare, explicability is essential; as it leads to higher rates of acceptance by users, who can thus fully learn how to optimise their use of these new tools by understanding their strengths and weaknesses in terms of their own expertise. For instance, when an AI solution says a CT scan shows signs of COVID-19 with 98% of confidence, but its projection is based on pixels outside the lungs, radiologists can easily ignore this result. In some other cases, AI programs may provide more reliable evidence and radiologists will take them into account to confirm or revise their initial diagnosis. Above all, AI must be used in the service of business or clinical decisions, rather than governing them.
How is the data which informs AI programs gathered? What measures are in place to ensure the security of users’ data?
PM: There are two ways to collect health data: pseudonymisation or anonymisation. Pseudonymisation is preferred when it is necessary to be able to re-identify a patient, for example to gather additional data. Authorisation is required and the research project must demonstrate why the requested data is necessary for the project. Anonymisation, on the other hand, must guarantee the absence of risk of re-identification by ensuring that there is no individualisation, no correlation and no inference. This involves some simple operations (deletion of name, date of birth and other key identifying markers) and some recoding operations (generating new and irreversible identifiers, sorting patients into age groups); but also some data analysis, to verify that the patient cannot be re-identified by cross-referencing the remaining data.
With regards to data security, French legislators introduced regulations in 2006 outlining requirements and best practices on the hosting of personal health data. Moreover, thanks to the GDPR, the Information Services branches of clinical and research facilities are widely aware of this subject; and check where and how patients’ data is transmitted to the companies which request it.
Is data interoperability between healthcare departments a significant factor in integrating AI technologies into healthcare? How can this be achieved?
JC: Interoperability is indeed the cornerstone for the transmission and consolidation of health information managed in Health Information Systems (HIS). In 1998, the Integrating Healthcare Enterprise (IHE) was created by the healthcare industry to organise itself, with the goal of collaboratively promoting and defining technical guidelines. The Health Level 7 (HL7) and Digital Imaging and Communications in Medicine (DICOM) standards provide a framework for exchanging structured data between various departments of one or many facilities. This obviously makes it much easier to gather data of a single patient coming from several departments or facilities.
Even if all areas of health do not yet have the same maturity level in terms of data, they are converging thanks to customer demand. To take the example of the medical imaging industry, which is highly industrialised, the integration of a computer-aided detection (CAD) result – such as mammography or pulmonology – is done using the DICOM standard. Thus, the CAD editor has only to define the overlays containing the marks to be displayed on the diagnostic console and the report without any particular need for additional integration. Therefore, the first step for startups is much lower than I started 20 years ago!
However, as the range of use cases becomes richer, standards must continue to be developed. This is why NEHS Digital and its AI partners are making a proposal to include AI results directly in the Research Information Systems (RIS), rather than having to display that additional information in an additional tool which is not convenient for the user (too many screens) and the AI partner (more developments to integrate). For example, it is more efficient that an AI service which analyses bone fractures can send its result directly to the RIS which can flag the patient record directly in the worklist of its main application, rather than show the results on another screen or tablet. It is faster and safer.
Finally, in the last few years, interoperability has begun to provide a higher level of interpretability of the data by adding a higher semantic level. To do this, shared dictionaries such as LOINC or ontologies like SNOMED CT are used in structure reports which follow the CDA-R2 standard (XML-based). There are two advantages of this: the first is that an AI algorithm can more easily ‘understand’ the meaning of a report and extract the information parts it needs; and the second is that AI algorithms can produce such reports more easily.
Interoperability is obviously significant for AI integration into healthcare – but be careful not to miss the right target! Although the current system allows access to a huge amount of data, it does not necessarily mean that the quality of this data is good; and interoperability alone cannot address this major point.
References
- The French Imaging Database Against Coronavirus (FIDAC) is an anonymised dataset composed of 8,200 series of CT-scans (3.5 million images in total), which will be available soon from the AI4EU European platform.
Patrick Malléa
Jérémy Clech
AI4EU
www.ai4eu.eu
This article is from issue 17 of Health Europa. Click here to get your free subscription today.